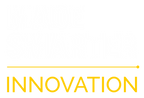
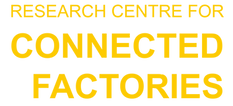
Background
The University of Sheffield research looks at production processes within factory workstations, particularly machining and assembly, to help make them more rapidly reconfigurable. To be competitive, production must adapt quickly to changes while maintaining high quality and minimising costs. The research is implemented in two primary technical areas: robotic machining and sensor-assisted assembly.
​
In the field of robotic machining, researchers focus on two key challenges. The first challenge is achieving geometric precision with highly flexible robotic machining systems that can handle frequent reconfiguration. New ways of generating and analysing data from industrial robotic platforms can help them achieve order-of-magnitude improvements in pose/position accuracy. To address this, a data-driven approach is used to adapt the robot controller through machine learning, resulting in a system that demonstrates precise machining operations across the entire workspace of the robotic system. The second challenge within robotic machining is extracting actionable insights from in-process sensor data, which is complicated by production variations and process noise. By employing deep learning techniques that optimise existing datasets and work effectively with minimal labelled data, the research moves away from traditional big data requirements. This has led to further development opportunities through Innovate UK funding – currently focused on multi-material stack drilling – with the deployment of the first deep learning models at production sites anticipated in the very near future.
​
For sensor-assisted assembly, the university has developed sensorised jigs designed to reduce setup times in reconfigured production processes. The challenge here lies in creating low-cost instrumentation and automation for production jigs and fixtures. To meet this challenge, cost-effective automation technologies are integrated with the latest machine vision algorithms to maintain precise assembly despite frequent reconfiguration and variations in the process. This work has received further support from ATI funding to focus on measurement-assisted precision assembly of large aerospace mechanisms. Additionally, the research has led to the development of novel sensors that improve robot awareness of human operators at a low-cost.
​
Another significant area of research is the development of new through-skin sensing techniques. Large, multi-material assemblies often develop geometric variations, such as gaps, that traditionally require partial disassembly to detect, increasing production lead times. To address this, researchers have created a new, human-safe technique that uses millimeter-wave electromagnetic signals capable of penetrating materials to extract key surface characteristics, thereby enabling non-invasive quality control and reducing production delays.
​
Through these innovations, the University of Sheffield is pioneering advanced, adaptable manufacturing processes that promise to make production systems more efficient, responsive, and cost-effective.
​
Demonstrators for Rapidly Configurable Machining Processes
​
The University of Sheffield has developed a range of demonstrators to showcase these innovations, supporting efficient, high-quality production in rapidly reconfigurable manufacturing environments. Each demonstrator highlights distinct technical advancements in robotic machining, sensor-assisted assembly, and novel sensing technologies to address common challenges in precision and adaptability. The demonstrators underscore practical applications of machine learning, sensor integration, and new sensing methods.
​
1. Robotic Drilling Demonstrator
​
-
Objective: Demonstrate precision robotic drilling within a reconfigurable setup.
-
Technical Focus: Employs a highly flexible robotic machining system capable of precise operation across varying workspace configurations. The system leverages a data-driven approach, utilising sensor data and machine learning to adapt the robot controller to geometric and operational changes.
-
Key Challenges Addressed:
-
Achieving geometric precision despite reconfiguration.
-
Mitigating effects of variability in the machining environment.
-
-
Outcomes: The robotic drilling demonstrator highlights successful control adaptations that enhance accuracy in placement and drilling depth, with potential applications across aerospace, automotive, and other high-precision manufacturing fields.
​
2. Mobile Robot Assembly Demonstrator
​
-
Objective: Enable flexible, sensor-assisted assembly processes using mobile robotics.
-
Technical Focus: Utilises sensorised jigs and advanced machine vision carried on mobile robot platforms to streamline and automate assembly tasks, even under varying production configurations. This setup focuses on achieving accurate assembly alignment and component fitment while reducing setup time and handling costs.
-
Key Challenges Addressed:
-
Integrating low-cost, effective sensing and vision systems.
-
Ensuring precision in reconfigured assembly processes.
-
-
Outcomes: The mobile robot assembly demonstrator showcases assembly of large aerospace structures with enhanced speed and accuracy. Notably, it features sensors that improve operator safety and awareness, even in tight working spaces, making it valuable for industries requiring frequent reconfiguration.
​
3. Through-Skin Sensing
​
-
Objective: Develop a method to inspect internal assembly features in multi-material structures without disassembly.
-
Technical Focus: Introduces "through-skin" sensing technology using millimetre-wave electromagnetic signals that penetrate surface materials to detect subsurface characteristics like gaps or misalignments. This technique is engineered to be human-safe, providing an innovative approach to non-invasive quality control in manufacturing.
-
Key Challenges Addressed:
-
Detecting hidden geometric variations without disassembly.
-
Reducing lead times and the need for intrusive inspections.
-
-
Outcomes: The through-skin sensing demonstrator allows for quick, precise identification of structural inconsistencies within assembled parts, reducing production delays. This is particularly beneficial for complex multi-material structures common in industries like aerospace and automotive, where traditional inspection methods can be time-consuming and invasive.
​
These demonstrators underscore the potential for cutting-edge manufacturing processes that are adaptive, precise, and resource-efficient, offering insights into the next generation of automated, sensor-driven production systems.
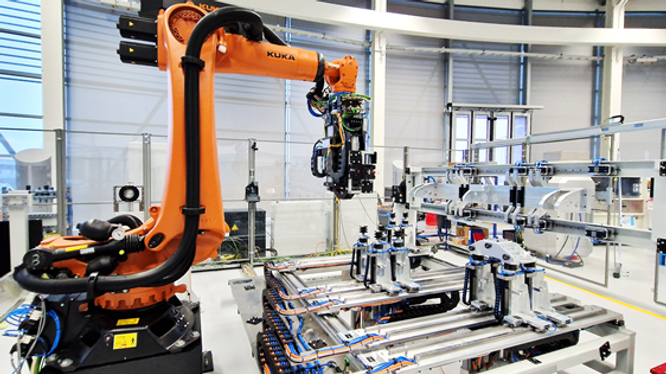